Pulse
Golden Whale Productions: the power of positive reinforcement
By William - 28 November 2023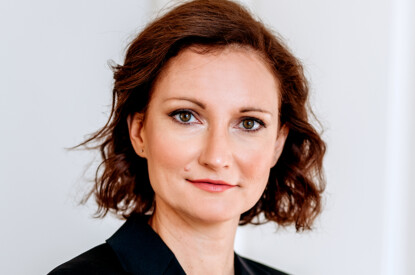
Golden Whale Productions’ Co-Founder and COO, Claudia Heiling, discusses how combining reinforcement-based systems with machine learning technology enables CRM teams to gain insights about their customers in a fraction of the time it takes to do manual A/B testing.
What impact can reinforcement-based systems have on CRM activity? How do these models utilise user data to test hypotheses and refine assumptions over time?
To answer this question in a general way we can say that Machine Learning Models always find useful applications whenever the problems are multidimensional, as these are most commonly the areas where humans have a difficult time comprehending the correlations.
Tiresome A/B testing can be substituted for experimental runs of our LOOPS systems, with optimised results being achieved far quicker and with less friction in the organisation.
For work in CRM, this means that the organisations using our methods can run more campaigns, add more new features and do more experiments each time while still creating better results due to the much-improved turnaround time for a singular event.
These systems don’t invent actions by themselves, but rather provide an accurate overview of current user behaviour that CRM teams can react to with ideas of their own. Can you give an example of a specific scenario a CRM manager may want to test based on the findings of their reinforcement system?
We have already established a very direct example that has an immediate impact on a business’s bottom-line with our Bonus Analytics, which is the question of when to give whom which bonus/feature on a platform level and within the boundaries of regulation.
This is an incredibly tricky optimisation problem for a human operator to solve on their own, but by running it through LOOPS we have managed to create monetisation uplifts of up to 30 per cent that CRM teams have been immediately able to utilise.
Furthermore, having the ability to identify even the most complex patterns and trends in user behaviour through LOOPS has enabled certain operators to shave up to 20 per cent off their bonus costs simply by allowing them to sort out the unproductive bonus targets from the ones that are likely to produce a longer-term yield.
Of course, the optimised execution speed of putting these questions through LOOPS has also sped up the learning cycles by weeks in each case, which has in turn allowed CRM teams to deploy the suggested strategies and reap the benefits of them faster than ever before.
How do you see the role of a CRM manager changing as this technology becomes more commonplace? Will there now be an even greater onus on CRM teams to have strong data analysis skills and creative problem-solving ability?
This is the most interesting change for me. As far as I see it, the scenario with this technology in place sees the CRM team lose some of the burden of having to run lengthy test cycles, which in turn allows it to focus more on ideation about what the actionable items should be that the system is offering to the user. The reinforcement system then does the testing and finds the sweet spot for the very scenario that they’ve created.
From there, it’s up to the CRM team to keep innovating and keep the players interested by finding more creative approaches to engagement. I see this as a far more satisfying approach to the process and a by far more interesting learning curve for everybody involved!
Another advantage of reinforcement-based systems is they can be combined with machine learning technology to create an iterative loop where self-inflicted changes are made to the data automatically. Can you explain how this process works in more detail?
At Golden Whale, we’ve made this process very straightforward. The moment you release a new model into our LOOPS system, the results of its actions begin to change the users’ experience and behaviour on your platform. This consequently creates a changed data stream that flows back into the model orchestration part of our system.
Here the changes are analysed, and the model might be adapted, re-calibrated or re-trained according to the impact made before, which in turn creates changes in the resulting data during the next round and so on and so forth. This is a very interesting process, and we are still improving how we automate and speed up the progress made through these logical iterations.
Teams will now be able to be far more proactive in their efforts to engage with customers and try new things rather than merely reacting to them after they’ve happened. How do you see this benefitting the customer experience going forward?
This is definitely a very important point. With the predictive part of our system, we get an educated guess about the future behaviour on the level of single users. This means that in the long run, we may even find ourselves in a position where we can work with a customer’s needs before he or she has made a conscious decision about something!
Being that much ahead of the curve will produce a new generation of products that cater to the demand of the customers in a way that’s never been seen before, ultimately resulting in an incredibly personalised user experience that can potentially be completely different from customer to customer.
This can, of course, only be of huge benefit from an engagement point of view and should open up plenty of new and interesting avenues for CRM teams.